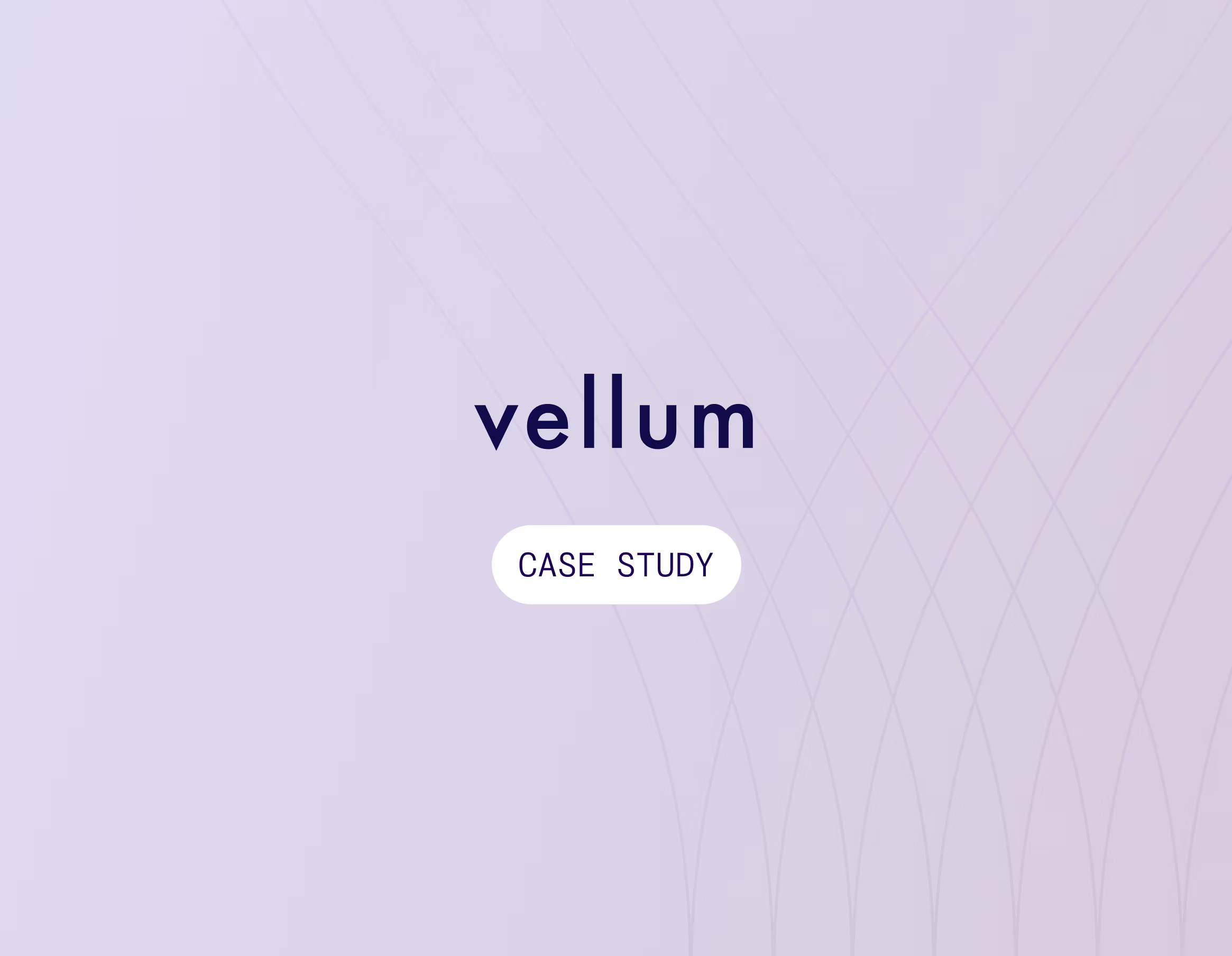
Privacy notice
In a world overflowing with information, students crave clarity and relevance.
They’re looking for study materials that actually work—materials that help them walk into exams feeling ready to succeed and gives them the confidence to participate actively in class discussions.
That’s why thousands of high school and college students turn to this ed tech company’s curated study guides to enhance their learning journey.
But, with colleges offering anywhere from 500 to several thousand classes across various fields, organizing all this content is a big task.
So naturally, this ed tech company wanted to scale their efforts using AI. But, creating high-quality AI-generated guides was no easy task. The team had to figure out:
• How to use AI to create these guides at scale while upholding standards?
• How to involve the entire team—teachers, writers, reviewers, engineers, and evaluators—to ensure these guides are accurate and effective?
They hit a classic AI development challenge: engineering became a bottleneck in producing study guides, and subject matter experts couldn’t effectively contribute to the process.
They turned to Vellum to get the whole team involved and speed up their AI development. This shift improved their time-to-deployment by 10x and saved over 50 engineering hours each month. Now, they can focus on their core mission: helping students succeed with high-quality study guides.
We spoke with the CEO and a Product Engineer from the company to learn more—here’s their journey from segmented and slow AI development, to being on track to publish more than 1,000 study guides in just three months.
Before integrating Vellum into their workflow, their team faced several challenges with their AI-driven content generation process.
In their first attempt, they used single prompts to generate the guides, but this approach quickly proved to be very limiting. The AI models struggled to handle complex tasks within a single prompt, leading the team to recognize the need for prompt pipelines (also known as prompt chains).
The CEO described the situation: “We realized pretty quickly that single prompts weren’t going to cut it. We needed a way to maintain context across our content, and that’s when we started looking into more complex workflows.”
The team needed a way to handle prompt chaining, so they tried to build their own internal tool to support it. Below you can check the initial version that they created—sadly, this process was still very taxing to the entire team, especially the engineers.

Before Vellum, the company’s engineers were the biggest bottleneck.
Feedback loops were slow, and refining custom scripts took up a lot of time. Scaling the process was tough because managing output and making tweaks required heavy engineering effort. This made it clear that they needed a more efficient and scalable solution.
The Product Engineer adds: “Updating prompts and managing feedback loops was a constant juggling act. We were constantly chasing our tails, trying to ensure that our prompts were up-to-date and aligned with user feedback. It felt like an endless cycle of manual updates and patchwork solutions.”
Today, the company uses Vellum to run a wide range of workflows in production—about 15-20 in total—including study guides, glossaries on key terms, and even image generation. They’ve moved from basic single-prompt generation to more advanced, chained workflows that ensure context is maintained throughout the content creation process. They capture end user feedback in a Reddit style upvoting method, and pass those values to continuously improve their systems.
The Product Engineer adds, “This has saved my sanity. Now, everyone can participate and contribute their input.”
Just look at their first version—engineers were overwhelmed by juggling multiple terminals to execute and update everything. Imagine how daunting this was for the non-technical team.
.png)
Now, non-technical team members like the CEO, who didn’t have an engineering background before using Vellum, regularly engage in prompt engineering and workflow creation. She adds: “I learned more about engineering this summer because of using Vellum. It made me feel very empowered.”
The Vellum UI allowed the company to parallelize their AI development. Non-technical team members handled the initial setup, and took the system from 0 to 1.
Once these workflows are validated and refined by the non-technical team, engineers step in to operationalize them. This involves adding retry logic to ensure the workflows are robust and can handle various edge cases without failure. Engineers also connect these workflows to the company’s servers, ensuring that the content generated by Vellum is seamlessly published and accessible to students.
The Product Engineer elaborated on this process: “After the non-technical team has validated a workflow, we come in to add the technical layers that make it production-ready. This includes retry logic, error handling, and integration with our backend systems. It’s a great system because it allows us to focus on optimizing and scaling rather than getting bogged down in the initial setup.”
This approach has significantly streamlined the company’s content production process, allowing them to create and publish high-quality study materials at a scale that would have been impossible before.
Adopting a generalist mindset and leveraging the right tools can transform AI development from a slow, bottlenecked process into a streamlined, efficient operation. This ed tech company’s experience with Vellum illustrates how these changes can dramatically speed up development and enhance team dynamics.
Total of 1000 Study Guides in just 3 months
Vellum’s user-friendly interface and robust features have enabled the team to move quickly and efficiently, testing new ideas and refining their workflows with minimal downtime. “We’ve been able to experiment with different workflows and features much more quickly than before,” the Product Engineer mentioned.
This summer, the team grew their collection of study guides from hundreds to thousands, covering both high school and college levels.
The CEO also highlighted this transformation: "We've seen a 100x increase in our product velocity using AI, and we've accelerated AI development by 10x using Vellum in the last three months. It's incredible how fast we can move now."
The Zipper effect
The introduction of Vellum has fundamentally changed how the company’s teams collaborate. The platform has blurred the lines between technical and non-technical roles, creating a more integrated and efficient working ecosystem.
The CEO emphasized this shift, saying, “We’re all becoming generalists. The collaboration between our teams is now seamless, and it feels like a zipper—everyone is aligned, everyone gets to work together, and everyone is a bit more technical.”
Everyone is a bit more technical
Vellum has empowered non-technical team members at this ed tech company to become more involved in the technical aspects of their work.
The CEO, who had no engineering background before using Vellum, now regularly engages in prompt engineering and workflow creation. “Vellum made it very empowering for me,” she said. “I wasn’t a coding person, but now I know what a JSON is. I’ve learned so much about engineering this summer because of Vellum.”
This empowerment has not only increased the team’s overall productivity but has also fostered a culture of continuous learning and growth.
This ed tech company’s experience with Vellum underscores the transformative potential of a robust AI development platform.
Engineering teams shouldn’t be the bottleneck of your AI initiatives—you can move much faster if you enable the right teams with the right tooling to enable parallel work, fast prompt iterations, and continuous improvements once in production.
If you’re looking to enhance your AI projects with quicker development cycles and better team synergy, Vellum is the tool you need - Get in touch today!
Latest AI news, tips, and techniques
Specific tips for Your AI use cases
No spam
Each issue is packed with valuable resources, tools, and insights that help us stay ahead in AI development. We've discovered strategies and frameworks that boosted our efficiency by 30%, making it a must-read for anyone in the field.
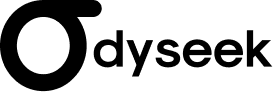
This is just a great newsletter. The content is so helpful, even when I’m busy I read them.

Experiment, Evaluate, Deploy, Repeat.
AI development doesn’t end once you've defined your system. Learn how Vellum helps you manage the entire AI development lifecycle.
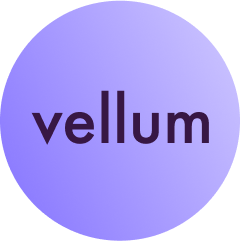