Learn how to use retrieval and content generation metrics to consistently evaluate and improve your RAG system.
Retrieval Augmented Generation (RAG) is a powerful technique that enhances output quality by retrieving relevant context from an external vector database.
However, building and evaluating a RAG system can be challenging, especially when it comes to measuring performance.
In this post, we'll explore the most effective metrics for each stage of your RAG pipeline and how to use them to evaluate your whole system.
When evaluating your RAG, you're essentially checking how effectively your system retrieves relevant information from a knowledge base and uses it to produce reliable and precise responses or content.
Running these evaluations are very useful when you’re building your first RAG version; but the benefit of running these evaluations continue post-development. Running these evals in production will help you understand your system's current performance relative to the potential improvements you could achieve by modifying your prompts.
It’s a never ending process, and without doing it, there’s no way to know if your RAG system is performing optimally or needs adjustments.
But, how to actually do it?
.png)
When evaluating your RAG system, you should pressure test the two most important parts: retrieval and content generation. However, don't overlook the significance of measuring all other aspects of your RAG that contribute to the underlying business logic of your system.
So, what exactly are we evaluating? Let's break it down:
1.Context retrieval
When you’re evaluating your “Context Retrieval” segment you’re essentially trying to figure out whether you can consistently retrieve the most relevant knowledge from a large corpus of text based on the optimal combination of chunking strategy, embedding model, and search algorithm.
2. Content generation
Evaluating the quality of generated content is basically running experiments with various prompts and models, with a goal of using metrics such as faithfulness/relevancy to determine that, given the most relevant retrieved knowledge, you produce a reasonable generated answer.
3. Business logic
The first two are must-haves. However, keep in mind that you should evaluate other parts of your AI workflow, that are important for your use-case and business logic. Intent verification, output length, rule compliance are some of the many metrics that businesses are using to evaluate important segments in their RAG pipelines.
To perform some of these evaluations you’ll either need human-annotated ground truth data to compare against, or you can use another LLM to synthetically generate that data for you, or you can evaluate your outputs on the spot (GPT-4 is very capable for this task, and it’s already widely used in the NLP community).
Now let’s look at the metrics that you should care about when performing these evaluations.
Finding the best ways to measure success for your RAG systems is forever changing and an active field of research. But there are some metrics that are proving to be useful for production-grade AI apps.
Context Retrieval Evaluation
To evaluate which retrieval setup produces the best results, you can use the following evaluators:
Context relevance
- How relevant is the context to the question?Context adherence
- Are the generated answers based on the retrieved context and nothing else ?Context recall
Content Generation Evaluation
Once you have a good semantic search process, you can start testing different prompts and models. Here are some frequent evaluation metrics:
Answer Relevancy
: How relevant is the answer to the question at hand?
For example, if you ask: “What are the ingredients in a peanut butter and jelly sandwich and how do you make it?" and the answer is "You need peanut butter for a peanut butter and jelly sandwich," this answer would have low relevancy. It only provides part of the needed ingredients and doesn't explain how to make the sandwich.
Faithfulness
: How factually accurate is the answer given the context?
You can mark an answer as faithful if all the claims that are made in the answer can be inferred from the given context. This can be evaluated on a (0,1) scale, where 1 is high faithfulness-
Correctness
: How accurate is the answer against the ground truth data? -
Semantic similarity
: How closely does the answer match the context in terms of meaning (semantics)?
These are just a few examples, but remember, there are many different methods to evaluate your RAG systems based on what's important for your business.
The key is to create a testing process to measure the system's effectiveness and reliability before it goes live. Additionally, once real users interact with it, you can gather their feedback to enhance the system by applying the same testing methods.
To do this best, you need the right tooling - and that's where we can help.
Using Vellum, you can create custom evaluators to evaluate every step in your RAG system. On top of that, our Evaluation Reports enables you to look at absolute and relative performance across various metrics like mean, median, p10 and p90.
.png)
For instance, when creating a customer support chatbot using Vellum's Workflow builder, you can set up all required RAG steps, and the evaluation mechanisms:
- Search: Initialize the vector database;
- Retrieval Evaluators: Create custom evaluators to check how accurately the chatbot retrieves context;
- Content Evaluators: Test out various prompts and models, including every model available, whether it's proprietary or open-source;
- Business logic eval: Create evaluators for business logic; build them scratch or use LLM-based evaluation when needed.
- Deploy: Launch your chatbot in production and capture implicit/explicit end user feedback. Then use this feedback as your baseline data to further evaluate your system;
- Continuously improve: Regularly conduct evaluation reports to ensure ongoing trust in your RAG system.
If you’d like to learn more on how to build this with Vellum, book a call here, or reach out to us at support@vellum.ai.
Latest AI news, tips, and techniques
Specific tips for Your AI use cases
No spam
Each issue is packed with valuable resources, tools, and insights that help us stay ahead in AI development. We've discovered strategies and frameworks that boosted our efficiency by 30%, making it a must-read for anyone in the field.
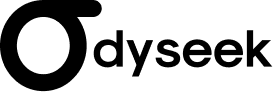
This is just a great newsletter. The content is so helpful, even when I’m busy I read them.

Experiment, Evaluate, Deploy, Repeat.
AI development doesn’t end once you've defined your system. Learn how Vellum helps you manage the entire AI development lifecycle.
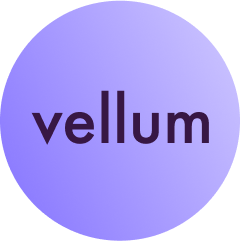