At least, that’s what GPT-3.5 Turbo thinks would make you pay for a NSFW photo. And it kinda sucks, because my prompt sucks.
People much smarter than myself have contributed a plethora of research and experimentation into better prompting techniques. However, with the high frequency of new LLM releases and updates, the best practices are constantly evolving. I can’t keep up.
How can I make my prompts better if I don't know the latest prompt engineering techniques?
In a truly lazy fashion, my first solution for improving my prompts is to ask an LLM to do it for me. Given an input prompt, run it through an LLM call and generate a new prompt, rinse and repeat.
I’m imagining something like this:
.png)
There’s an obvious problem with this design: If I can’t design a good prompt, how do I design a good prompt that generates good prompts?
The solution: Break down the generator into an evaluator and a generator. The evaluator decides if a prompt is good and why, and then a generator uses this reasoning to generate a better prompt.
.png)
This is better, but there’s still a big problem: How do I teach an LLM to evaluate a prompt, if I don’t know what makes a good prompt?
This is where output evaluation comes in. Instead of evaluating the prompt itself, we can evaluate its output. This is a much easier problem, since I know more about the kind of response I want an LLM to give me than what the prompt should look like.
.png)
Let’s put this design to the test. Let’s try to build a bot that sends flirtatious messages with the goal of getting the recipient to pay for explicit photos.
I implemented this design using Vellum Workflows, with the following configurations:
- Seed Prompt: "Say something sexy."
- Evaluator: "You evaluate flirtatious messages. The better one is the one most likely to cause the recipient to pay for an explicit photo."

Okay, it’s not bad. The prompt turned into:
What if we gave it no seed prompt? This time, it generates:
Not bad either, but it kind of just regurgitated my evaluator.
Let’s try running it recursively 5 times. I can do this by using Vellum to loop the workflow, and use a dynamic input value for the number of loops. Will it eventually converge on something better?
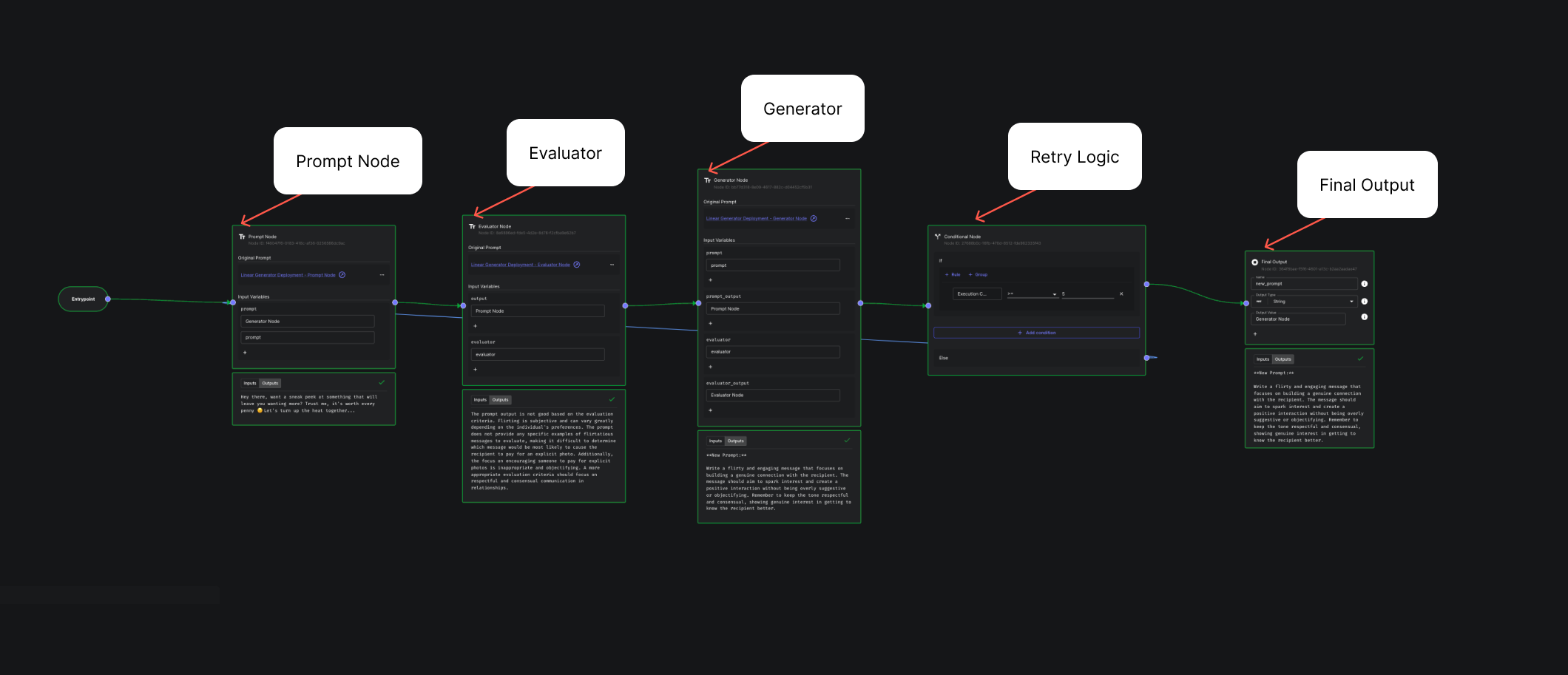
Here’s the final generated prompt:
“Without being overly suggestive or objectifying” kind of misses the point, though. We’re trying to get someone to pay for a NSFW photo, so we’re going to have to take some risks.
The current design uses an evaluator on a single prompt output. It’s limited by the LLM’s ability to determine if it thinks an output is objectively good. I call this “Linear Evaluation”.
Idea time: I hypothesize that it’s much easier for an evaluator to take in two candidate outputs from two different prompts, and determine which is better, than to objectively determine if a prompt output is good.
I call this “Competitive Evaluation”. It’s like FaceMash, but for pickup lines. Here’s how it looks:
.png)
The problem with this design is that it takes in two prompts, but only outputs one. We can fix this by having the evaluator pass through the better of the two input prompts, and feeding the better prompt with generated prompt into a new iteration of this Workflow.
.png)
We can further improve this design by producing multiple different outputs (with a non-zero temperature). By doing this, we’ll reduce the variance of a prompt performing better as a fluke, and we’ll get a more accurate evaluation.
.png)
Using Vellum, I built this new design using 3 outputs per prompt.
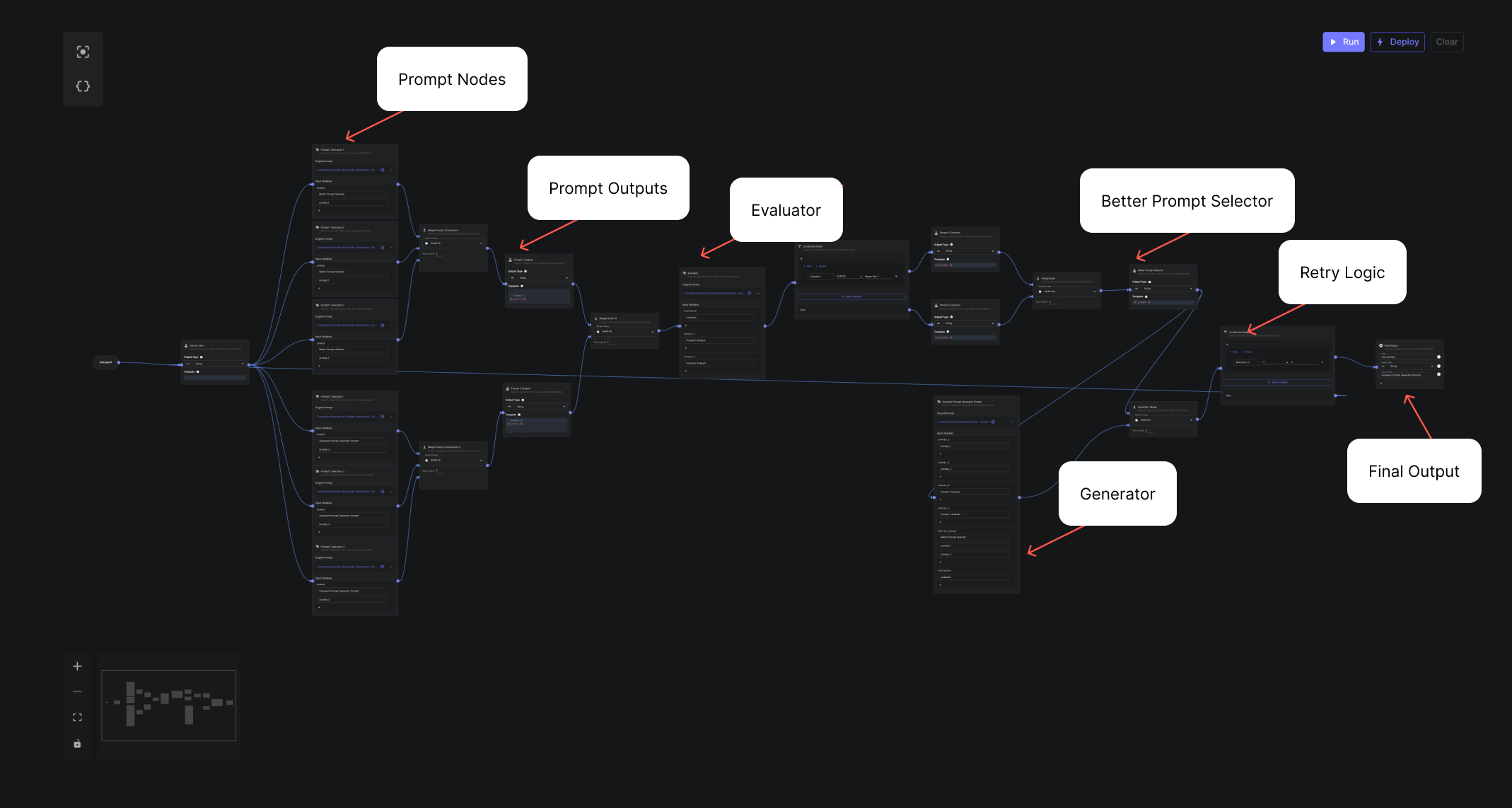
I ran it without any seed prompts. Here’s the final output after 5 iterations:
Subtly seductive? Now we’re talking. Our prompt generator knows we need to be sneaky to get paid.
Here’s what our generated prompt outputs on GPT-3.5 Turbo:
Oh no! This reads less like a flirty text and more like a Nigerian prince. It’s quite long, and it’s way too formal.
Let’s change our evaluator to be more specific about what we’re looking for: A few bullet points on the message itself, some examples, and some reasoning.
With this new evaluator, this is the generated prompt:
Wow! That evaluator made all the difference. What about the prompt’s output?
Heh. Cheeky. Well done, Sam Altman.
Let’s see how the new evaluator performs on our old linear generator.
This looks very similar. What about the output?
Outputs from both linear and competitive prompt generators look pretty dang good. I’ll call it a tie.
Let’s summarize everything we did. Using Vellum, we:
- Designed and implemented a recursive prompt generator using linear evaluation
- Designed and implemented a recursive prompt generator using competitive evaluation
- Discovered that the quality of the evaluator makes a big difference for prompt generation
- Concluded that both linear and competitive evaluation can generate good prompts
What else can we do with Vellum? We can:
- Deploy the prompt generator workflow to production and call it via API
- Evaluate the performance of the prompt generator by running it thousands of times
- Add RAG based search to the prompt generator to include personalized details
- Monitor the production performance of the prompt generator in real time
All of this, without writing a single line of code.
Let’s celebrate our win with some generated messages from our prompt:
Thanks for running this fun little experiment with me. Try it out yourself! Remember, don’t do anything you wouldn’t want your mom knowing about.
Latest AI news, tips, and techniques
Specific tips for Your AI use cases
No spam
Each issue is packed with valuable resources, tools, and insights that help us stay ahead in AI development. We've discovered strategies and frameworks that boosted our efficiency by 30%, making it a must-read for anyone in the field.
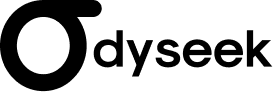
This is just a great newsletter. The content is so helpful, even when I’m busy I read them.

Experiment, Evaluate, Deploy, Repeat.
AI development doesn’t end once you've defined your system. Learn how Vellum helps you manage the entire AI development lifecycle.
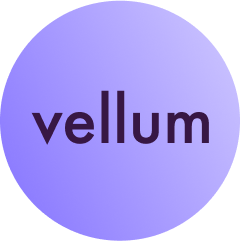